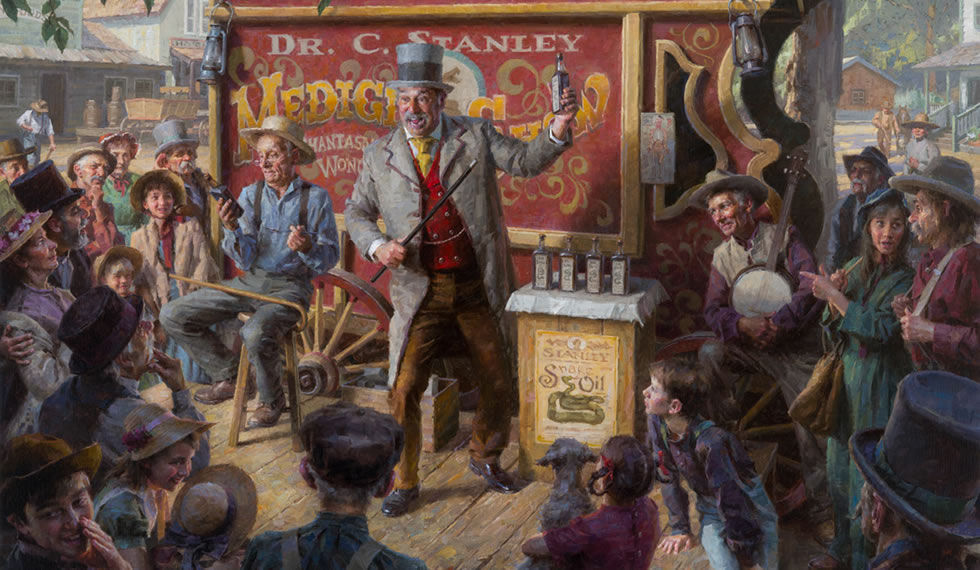
In the last few weeks I have been quite entertained by a book that seemed simple, but became a great ally in understanding the current moment of the Artificial Intelligence market, and the apparent madness we are living.
As a technology executive, it’s common to hear promises of tools that are presented as game-changing and that will disrupt the entire market when they reach their full potential. It’s my responsibility to be skeptical when analyzing the possibilities and to understand when we’re being misled. What every tool vendor wants is to maximize the use of their tools.
With AI, it could not be different. The big detail is that technology is anchored in our fantasies and deepest biases to gain space and not be questioned. Regardless of the countless controversies and almost no concrete evidence involving the use of machine learning for Facial Recognition , Crime Prediction or even life expectancy , many of us continue to believe in promises of superior intelligence. The impacts can be tragic.
Under these premises that Arvind Narayanan and Sayash Kapoo , Princeton academics, present this year the book AI Snake Oil , with the proposal to discuss what really works in artificial intelligence, and what is merely a marketing ploy and abuse of influence with often devastating consequences from companies aiming only to surf the current hype. Being two academics with experience also in large technology companies, the duo brings a blunt view on the subject.
The book is very good and highly recommended for anyone who wants to understand the current state of the artificial intelligence market in a direct and skeptical way, with hundreds of references to delve even deeper than the many pages of content that the book presents. The material delves into what AI has most to offer at the moment: the problems. Despite all the marketing behind companies that profit from selling tools, there are countless cases of errors, misuse and problems that no one wants to talk about.

"Artificial intelligence is an umbrella term for a set of loosely related technologies. ChatGPT has very little to do with the software banks use to profile a person for loans. Both are billed as AI, but for all intents and purposes, the way the tools work, who they are used by, and how they fail, could not be more different."
The more we look at the potential of machine learning, the more we come across real risks that, due to lack of knowledge or bad intentions on the part of companies, we are not giving due attention to.
One of the most important points of the book is to break down the broad term Artificial Intelligence into three themes that are usually lumped together but are completely independent. The results in Generative AI do not necessarily mean that any predictive system using machine learning will work.
Knowing how to separate solutions is the best way to increase the results of using tools, and also knowing when not to use them. Here it is important to say that I am quite critical of technology and its prospects for high-risk and high-impact use, given that the implementation of AI tools is driven more by vision than by necessity.
"By presenting technology as super-powerful, critics exaggerate its capabilities while downplaying its limitations, favoring companies that will always benefit from the lack of scrutiny of their products."
There are three main themes that the book addresses:
Predictive AI - The one that works the least
Quite directly, the book addresses the fact that most likely no predictive AI actually works. And it presents evidence of how companies often disguise the results of their tools and exaggerate their capabilities to gain more media coverage and effectively speculate about the potential of their products.
Predictive AI is seductive because it makes decision-making more efficient, and it is precisely through efficiency that we lose accountability. Our own automation bias puts us in a position to blindly accept the results of a predictive system that is often no better than flipping a coin.
"The fundamental limitation of Predictive AI: It is possible to make some predictions if no information changes. But correlation is not causation."
In addition to countless cases of failures in the use of tools, which I intend to explore in another post, perhaps the best lesson here is:
The main error of prediction tools is to exaggerate the results because training data is almost always used to evaluate the accuracy of the systems. This is a common error that always generates inflated numbers, easily exploited by marketing teams. After all: what is the point of a prediction product that works as well as choosing randomly?
Five Reasons Why Predictive AI Fails
Reason | Example |
A good prediction can result in a bad decision. | Asthma patients may be sent home when they arrive at the hospital with symptoms of pneumonia. |
People can strategically manipulate opaque AI. | Adding bookshelves in the background increases scores in automated hiring tools. |
Users over-rely on AI without proper supervision or resourcing. | The Dutch welfare fraud detection model falsely accused 30,000 parents of fraud without any recourse. |
The data to train the AI may come from a different population than the one it is used on. | The PSA's crime risk prediction was based on a national sample. It overestimated risk in counties where crime was rarer. |
Predictive AI could increase inequality. | Optum’s Impact Pro has led to an increase in the gap in quality of care between Black and white patients. |
Generative AI - Benefits and Risks
Perhaps because it has been the main topic in technology in recent years, this is the topic in which there are the most cases of problems and benefits recorded today. The book seeks to present the challenges of using generative tools in the context of their real impact on society.
I've written a lot about this topic here, and it's worth following to expand the repertoire. From the absurd claim that LLMs will replace programmers , to the fact that there is no generative AI without unauthorized third-party intellectual property .
AI Content Moderator
Content moderation through AI is a very important topic for social networks. As a complex topic that crosses many cultural aspects of our existence, it is naive to believe that through AI alone we will be able to act correctly.
Suicide prevention, hate speech and copyright are all topics that have been addressed within this sphere, but they have been approached in completely different ways. In the end, platforms like YouTube have solved the problems of abuse of intellectual property content only because it has a direct financial impact on the company.
Content moderation at scale depends on people, and the cultural incompetence of large platforms has tragic impacts on the world, to the point that extreme acts of violence have been known to be influenced by social platforms, without the companies being held accountable.
Scientific reproducibility
Perhaps the most interesting point for me in the book is the fact that both authors investigated how current research using Machine Learning makes mistakes to the point of making it impossible for other scientists to reproduce it, a serious error that impedes the advancement of research in artificial intelligence.
A common mistake in AI is that many models are evaluated using the data used to train the models, which is known as Data Leakage. This causes exaggerated optimism in the results of a model, giving a false sense of success.
Well, they analyzed over 600 scientific papers and found data leakage issues in almost all of them, which invalidates any claims made in these papers!
"We reviewed the academic literature to find similar results to ours. It turned out that there was no shortage of errors due to leaks in AI-based science. Hundreds of papers across more than a dozen scientific disciplines—including medicine, psychiatry, computer security, IT, and genomics—had been affected by leaks. One of the papers with errors was actually co-authored by Arvind, showing that even researchers studying the limitations of AI can succumb to these errors."
The problem is so serious that there is even a website dedicated to such studies, to raise more awareness of the fact: https://reproducible.cs.princeton.edu
A very interesting book, and despite being long, it will become a reference in my studies.
I highly recommend the book for anyone who wants to delve deeper into the subject, and especially for anyone looking for a more skeptical view of the benefits of AI for the general public.
Comments